by Huenei IT Services | Apr 27, 2021 | Infra, Process & Management
The article Key Concepts about Data Lakes delved into the importance of Data Lakes, their architecture and how they compare to Data Warehouses. This article will focus on deployment using
Amazon Web Services (AWS), Amazon’s cloud platform. We will look into the
overall flow, the different
services available and, finally,
AWS Lake Formation, a tool specially designed to facilitate this task.
Overall Flow
Data Lakes support the needs of our applications and analytics, without the need to constantly worry about increasing storage and computing resources as the business grows and the data volume increases. However, there is no magic formula creating them. Generally, they involve dozens of technologies, tools and environments. The diagram below shows the overall flow of data, from collection, storage and processing, to the use of analytics via Machine Learning and Business Intelligence techniques.
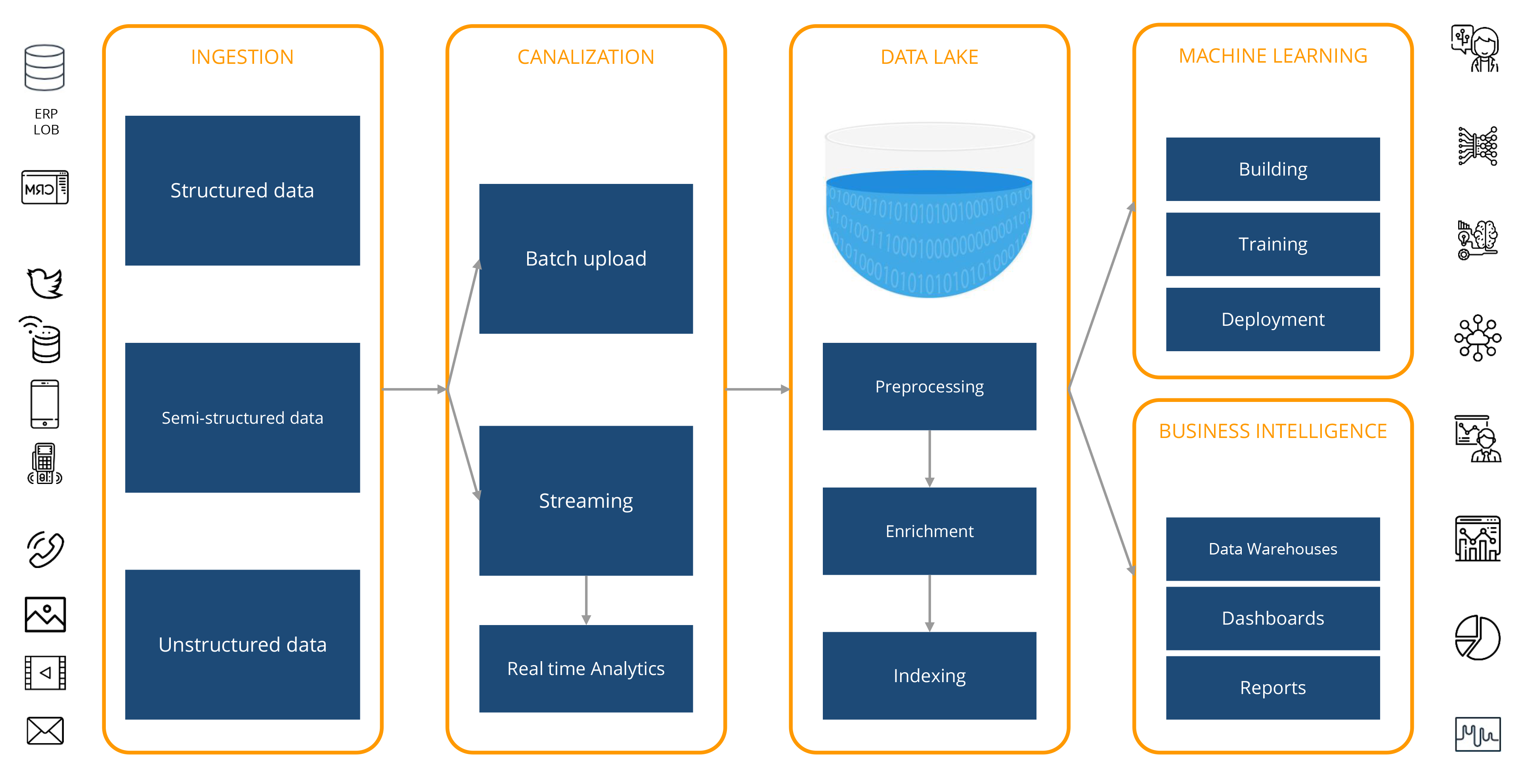
Services supported by AWS
AWS provides a comprehensive set of managed services that help build Data Lakes. Proper planning and design are necessary to migrate a data ecosystem to the Cloud, and understanding Amazon’s offerings is critical. Below are only a few of the most important tools at each stage of the flow.
Collection
The first step is to analyze the goals and benefits you want to achieve with the implementation of an AWS-based Data Lake. Once the plan is designed, data must be migrated to the Cloud, taking into account its volume. You can easily accelerate this migration with services such as Snowball and Snowcone (edge devices for storage and computing) or DataSync and Transfer Family, to simplify and automate transfers.
Channeling
In this step, you can operate in 2 modes: Batch or Streaming.
In Batch Loading, AWS Glue is used to extract information from different sources, at periodic intervals, and move them into the Data Lake. It usually involves some degree of minimal transformation (ELT), such as compression or data aggregation.
For Streaming, data generated continuously from multiple sources, such as logging files, telemetry, mobile applications, IoT sensors and social networks, are collected. They can be processed during a circular time window and channeled into the Data Lake.
Real-time analytics provides useful information for critical business processes that rely on streaming data analysis, such as Machine Learning algorithms for anomaly detection. Amazon Kinesis Data Firehose helps perform this process from hundreds of thousands of sources in real time, rather than uploading data for hours and processing it at a later stage.
Storage and Processing
The core service in any AWS Data Lake is Amazon S3, which provides high scalability storage, excellent costs and security levels, thus offering a comprehensive solution for different processing models. It can store unlimited data and any type of file as an object. It allows you to create logical tables and hierarchies from folders (for example, by year, month, and day), allowing the partition of data in volume. It also offers a wide set of security functions, such as access controls and policies, encryption at rest, registration, monitoring, among others. Once the data is uploaded, it can be used anytime, anywhere, to address any need. The service supports a wide range of storage classes (Standard, Smart, Rare Access), each with different capacities, recovery times, security and cost.
AWS Glacier is a service for secure archiving and backup management at a fraction of the cost of S3. File recoveries can take from a few minutes to 12 hours, depending on the storage class selected.
AWS Glue is a managed ETL and Data Catalog service that helps find and catalog metadata for faster queries and searches. Once Glue points to the data stored in S3, it analyzes it using automatic trackers and records its schemes. Glue is designed to perform transformations (ETL/ELT) using Apache Spark, Python scripts and Scala. Glue has no server; therefore, there is no infrastructure configured, which makes it more efficient.
If the contents of Data Lake need to be indexed, AWS DynamoDB (NoSQL database) and AWS ElasticSearch (text search server) can be used. In addition, by using AWS Lambda features, activated directly by S3 in response to events such as uploading new files, processes can be triggered to keep your Catalog up to date.
Analytics for Machine Learning and Business Intelligence
There are several options for massive Data Lake information.
Once data has been catalogued by Glue, different services can be used in the client layer for analytics, visualizations, dashboards, etc. Some of these are Amazon Athena, an interactive serverless service for ad hoc exploratory queries using standard SQL; Amazon Redshift, a Data Warehouse service for more structured queries and reports; Amazon EMR (Amazon Elastic MapReduce), a managed system for Big Data processing tools such as Apache Hadoop, Spark, Flink, among others; and Amazon SageMaker, a Machine Learning platform that allows developers to create, train and implement Machine Learning models in the cloud.
With Athena and Redshift Spectrum, you can directly query the Data Lake in S3 using the SQL language in the AWS Glue Catalog, which contains metadata (logical tables, schemes, versions, etc.). The most important aspect is that you only pay for the queries executed, depending on the scanned data volume. Therefore, you can achieve significant performance and cost improvements by compressing, partitioning, or converting data into a column format (such as Apache Parquet), as each of those operations reduces the amount of data Athena or Redshift Spectrum should read.
AWS Lake Formation
Building a Data Lake is a complex, multi-step task, including:
- Identify sources (Databases, files, streams, transactions, etc.)
- Create the necessary buckets in S3 to store data with the applicable policies.
- Create the ETLs that will carry out the necessary transformations and the corresponding administration of audit policies and permits.
- Allow Analytics services to access Data Lake information.
AWS Lake Formation is an attractive option that allows users (both beginners and experts) to immediately start with a basic Data Lake, eliminating complex technical details. It allows real-time monitoring from a single point, without having to go through multiple services. One strong aspect is cost: AWS Lake Formation is free. You will only be charged for the services you invoke from it.
It allows loading from various sources, monitoring flows, configuring partitions, enabling encryption and key management, defining transformation jobs and monitoring, reorganizing data in column format, configuring access control, eliminating redundant data, relating linked records, gaining access and auditing access.
Conclusions
These 2 articles looked into the definition of Data Lakes, what makes them different from Data Warehouses and how they can be deployed on the Amazon platform. CTO can be significantly reduced by moving your data ecosystem to the cloud. Suppliers such as AWS add new services continuously, while improving existing ones and reducing costs.
Huenei can help you plan and execute your Data Lake initiative in AWS, in the process of migrating your data to the cloud and implementing the analytics tools necessary for your organization.
by Huenei IT Services | Mar 26, 2021 | Infra, Process & Management
Data has become a vital element for digital companies, and a key competitive advantage. However, the volume of data that organizations currently have to manage is very heterogeneous and its growth rate is exponential. This creates a need for storage and analysis solutions that offer scalability, speed and flexibility to help manage these massive data volumes. How can you store and access data quickly while maintaining cost effectiveness? A Data Lake is a modern answer to this problem.
This series of articles will look into the concept of Data Lakes, the benefits they provide, and how we can implement them through Amazon Web Services (AWS).
What is a Data Lake?
A Data Lake is a centralized storage repository that can store all types of structured or unstructured data at any scale in raw format until needed. When a business question arises, the relevant information can be obtained and different types of scans can be carried out through dashboards, visualizations, Big Data processing and machine learning to guide better decision-making.
A Data Lake can store data as is, without having to structure it first, with little or no processing, in its native formats, such as JSON, XML, CSV, or text. It can store file types: images, audio, video, weblogs, data from sensors, IoT devices, social networks, etc. Some file formats are better than others, such as Apache Parquet, which is a compressed column format that provides very efficient storage. Compression saves disk space and I/O access, while the format allows the query engine to scan only the relevant columns, reducing column time and costs.
Using a distributed file system (DFS), such as AWS S3, allows to store more data at a lower cost, providing multiple benefits:
- Data replication
- Very high availability
- Low costs at different price ranges and multiple types of storage depending on the recovery time (from immediate access to several hours)
- Retention policies, allowing to specify how long to keep data before it is automatically deleted
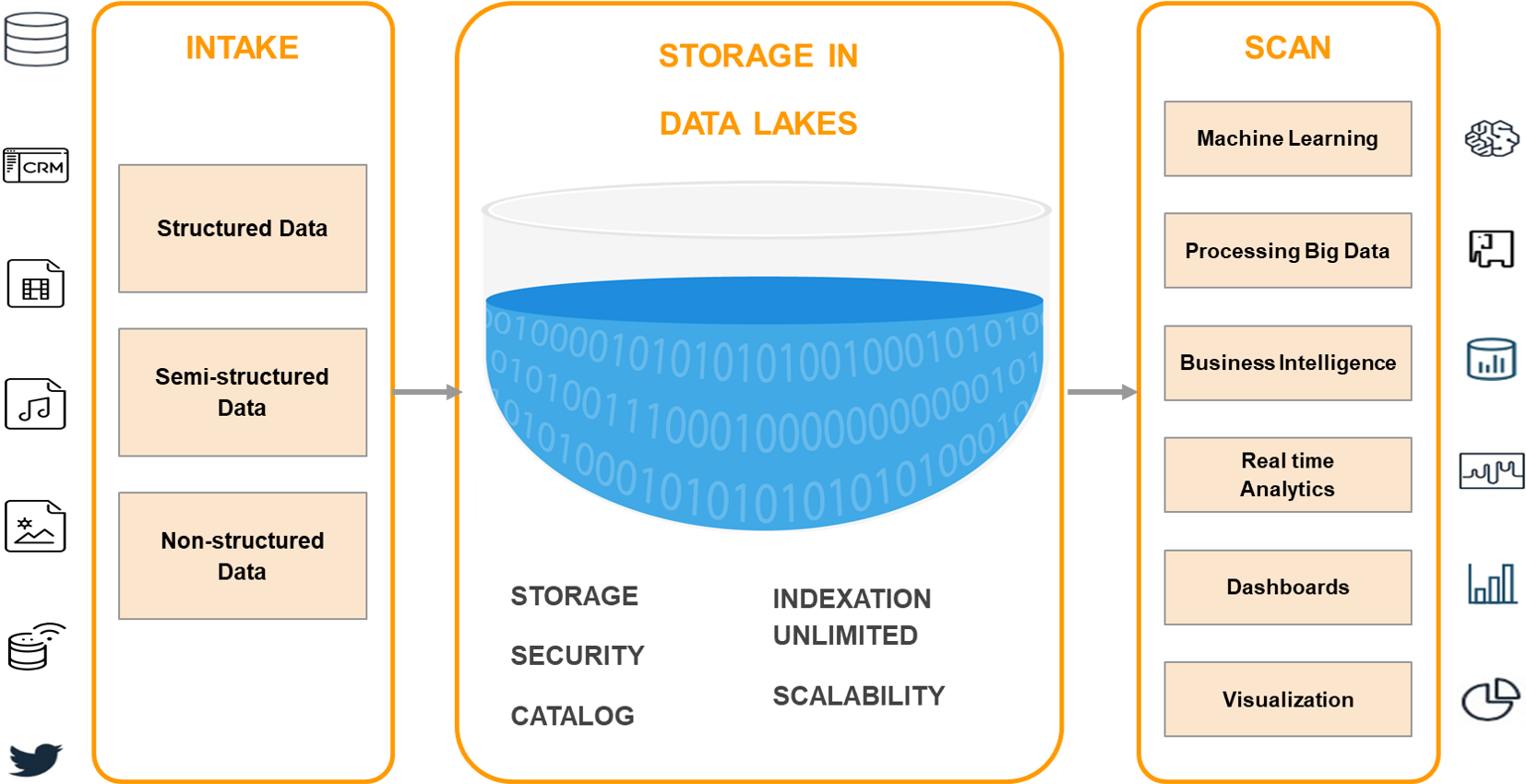
Data Lake versus Data Warehouse
Data Lakes and Data Warehouses are two different strategies for storing Big Data, in both cases without being tied to a specific technology. The main difference between them is that, in a Data Warehouse, the data scheme is pre-established; you must create a scheme and schedule your queries. Powered by multiple online transactional applications, data has to be converted via ETL (extract, transform and load) to conform to the predefined scheme in the data warehouse. In contrast, a Data Lake can host structured, semi-structured, and unstructured data and has no default scheme. Data is collected in its natural state, requires little or no processing when saved, and the scheme is created during reading to meet the processing needs of the organization.
Data Lakes are a more flexible solution adapted to users with more technical profiles, with advanced analytical needs, such as Data Scientists, since a level of skill is needed to be able to classify the large amount of raw data and easily extract its meaning. A data warehouse focuses more on Business Analytics users, to support business inquiries from specific internal groups (Sales, Marketing, etc.), by owning the data already curated and coming from the company’s operating systems. In turn, Data Lakes often receive both relational and non-relational data from IoT devices, social media, mobile apps, and corporate apps.
When it comes to data quality, Data Warehouses are highly curated, reliable, and considered the core version of the truth. On the other hand, Data Lakes are less reliable since data could come from any source in any condition, be it curated or not.
A Data Warehouse is a database optimized to analyze relational data, coming from transactional systems and business line applications. They are usually very expensive for large volumes of data, although they offer faster query times and higher performance. Data Lakes, by contrast, are designed with a low storage cost in mind.
Some of the legitimate criticism Data Lakes have received is:
- It is still an emerging technology compared to the strong maturity model of a Data Warehouse, which has been in the market for several years.
- Data Lakes could become a “swamp”. If an organization has poor management and governance practices, it can lose track of what exists at the “bottom” of the lake, causing it to deteriorate and making it uncontrolled and inaccessible.
Due to these differences, organizations can choose to use both a Data Warehouse and a Data Lake in a hybrid deployment. One possible reason would be adding new sources or using the Data Lake as a repository for everything that is no longer needed in the main data warehouse. Data Lakes are often an addition or evolution to an organization’s current data management structure rather than a replacement. Data Analysts can use more structured views of the data to get the answers they need and, at the same time, Data Science can “go to the lake” and work with all the raw information as necessary.
Data Lake Architecture
The physical architecture of a Data Lake may vary, since it is a strategy applicable by multiple technologies and providers (Hadoop, Amazon, Microsoft Azure, Google Cloud). However, there are 3 principles that make it stand out from other Big Data storage methods, and they make up its basic architecture:
- No data is rejected. They are loaded from multiple source systems and preserved.
- Data is stored in an untransformed or nearly untransformed condition, as received from the source.
- Data is transformed and a scheme is adapted during analysis.
While information is largely unstructured or geared to answering specific questions, it must be organized as to ensure that the Data Lake is functional and healthy. Some of these features include:
- Tags and/or metadata for classification, which can include type, content, usage scenarios, and groups of potential users.
- A hierarchy of files with naming conventions.
- An indexed and searchable Data Catalog.
Conclusions
Data Lakes are becoming increasingly important to business data strategies. They respond much better to today’s reality: much larger volumes and types of data, higher user expectations and a greater variety of analytics, both business and predictive. Both Data Warehouses and Data Lakes are intended to coexist with companies that want to base their decisions on data. Both are complementary, not substitute, and can help any business to better understand both markets and customers, as well as promote digital transformation efforts.
Our next article will delve into how we can use Amazon Web Services and its open, secure, scalable, and cost-effective infrastructure to build Data Lakes and analytics on top of them.
by Huenei IT Services | Jan 30, 2021 | Infra, Software development
Introduction
The revolution of Serverless Computing is here to stay, and this is because this new technology enables application development without having to go through the management and administration of a server. Under this model, applications can be grouped and loaded onto a platform and then run and scaled as demand for them increases.
Although “Serverless Computing” does not suppress the use of servers when executing a code, it does eliminate all activities related to its maintenance and updating. This creates an efficient model where developers manage to disassociate themselves from those routine tasks to focus on more productive activities, thus increasing the company’s operational efficiency.
What is Function as a Service (FaaS)?
Function as a Service (FaaS) is a model that allows for the execution of several computing actions based on events, and thanks to it, developers can manage applications, “bypassing” the need for servers during their management.
In the world of computing, functions are in charge of managing the states of a server, therefore the FaaS model develops a new logic that is later executed in other containers located in the cloud.
In general terms, FaaS allows us to design applications in a new architecture where the server works in the background and the execution of codes based on events becomes the fundamental pillar of the model. This means that the underlying processes that normally occur on a server do not run continuously, but are available when needed.
This becomes a clear advantage of the FaaS model, allowing developers to scale dynamically, that is, implement application automation so that it decreases or increases based on actual demand.
In addition to the above, FaaS increases the efficiency and profitability of operations, since providers will not bill the company when no activity is detected.
All this makes the FaaS model an innovative element within the recent field of serverless architecture by minimizing investment in infrastructure, and leveraging the competitive advantages of Cloud Computing.
The evolution of Serverless Computing
With the advent of the cloud in the first decade of the 2000s, people had the opportunity to store and transfer data online, which eliminated the need for hard drives.
This undoubtedly created important advantages for users, who had the opportunity to immediately access their information online from any device.
However, developers were missing an element in this equation, i.e., the place where applications or software were implemented. In this sense, a “Virtual Machine” model was implemented which allowed to point to a “Simulated Server”, creating significant flexibility in updates and migrations, and with this, the problems associated with hardware variations were left behind.
Despite this progress, “virtual machines” had some limitations in terms of operation, and this led to the creation of containers, a new technology that allowed administrators to section the operating system in order to keep several applications active simultaneously, without one interfering with the other.
Considering this reality, we can see that all these technologies maintain the paradigm of “where an application runs” as their fundamental structure. Under this scenario, Serverless Computing emerged, promising a new level of abstraction focused on the code itself that diminished the importance of the place where code was stored.
With the advent of Amazon’s AWS Lambda service at the end of 2014, a milestone in serverless architecture was achieved, as developers could finally focus their efforts on creating software without having to worry about hardware, OS maintenance, the location of the application, as well as its level of scalability.
Use Cases for Serverless Computing
Below are some successful cases of companies that applied serverless technology, or Serverless Computing, within their organizations:
Case 1. Major League Baseball Advanced Media (MLBAM)
Major League Baseball has used serverless computing technology to provide all its fans with real-time baseball game data through its “Statcast” product. This acquisition has increased MLBAM’s processing speed, as well as the ability to handle more data.
Case 2. T-Mobile US
T-Mobile US is a mobile phone company with a strong presence in the North American market. The company decided to bet on serverless technology, achieving significant benefits in terms of resource optimization, scaling simplicity, and the reduction of computer patches, thus increasing its real capacity to respond in a much more efficient way to all its customers.
Case 3. Autodesk
Autodesk is a company that develops software for the architecture, construction and engineering industries. Recently this organization decided to apply serverless technology in order to manage its development, as well as the time-to-market of all its products. In keeping with this policy, Autodesk created the “Tailor” application as an efficient response for managing its clients’ accounts.
Case 4. iRobot
iRobot is a company that designs and manufactures robotic devices intended for use within the home and in industrial settings. Since the organization decided to get involved with Serverless Computing technology, the data processing capacity of its robots has increased substantially, also allowing the capture of data streams in real time. The new serverless architecture allows them to focus on their customers and not on operations.
Case 5. Netflix
Netflix has become one of the world’s largest online media on-demand content providers. In line with its innovative spirit, this company has decided to use Serverless Computing to generate an architecture that helps optimize the encoding processes of its audiovisual files, as well as the monitoring of its resources.
Conclusions
When we look at the evolution of Serverless Computing and how it has managed to significantly impact computing processes in general, we understand that this new system will quickly become the next step in the world of cloud computing, fostering a promising future focused on adopting a multimodal operational approach.
by Huenei IT Services | Jan 15, 2021 | Infra, Software development
Introduction
Innovation in the world of computing occurs at a startling pace in each and every area, generating important progress in the processes related to “Serverless Computing”, also known as “Serverless Architecture”.
In this context, an increasing number of companies are turning to the “Cloud” as a way to optimize the creation and execution of applications and processes, minimizing the use of servers. This is where Serverless Computing comes in as a key element for the proper development of internal software architecture.
Although Serverless Computing reduces the use of a server, the server does not disappear in its entirety; it is simply optimized and reassigned by the cloud provider, who will ultimately be responsible for all the routine activities associated with the servers’ maintenance.
Background
In the beginning, creating a web application required the use of hardware that would allow the execution of a server, sometimes resulting in a complicated and expensive process. Later on, when the cloud came along, companies and developers had the possibility to rent spaces on remote servers to carry out their activities.
However, this process was not entirely efficient either, since companies ended up buying more space than necessary in order to ensure the system would remain stable in case of very high demand peaks, thus incurring in additional expenses. This is why developers began to see the need for a platform that would allow them to pay only for the space used.
In this sense, the story of Serverless Computing is recent, the first reports of this technology being found in an article by the specialist in decentralized applications and serverless development, Ken Fromm, published in October 2012, titled “Why the Future of Software and Apps is Serverless.”
By November 2014, the Amazon company launched its “AWS Lambda” service, which allows developers to execute code and automatically organize resources without the need to manage the underlying infrastructure during.
A year later, in July 2015, Amazon created “API Gateway”, a service for the creation and maintenance of API REST, HTTP and WebSocket, where developers can generate Application Programming Interfaces that access Amazon or other Web Services, as well as data stored in the cloud. Finally, in October 2015, “Serverless Framework” was born as the first framework developed for creating applications on AWS Lambda.
Serverless architecture overview
Serverless Computing, or serverless architecture, does not imply the total absence of a server as such; what this system actually seeks is for the cloud provider to adequately and efficiently manage all processes related to the server.
In this sense, one of the outstanding features of Serverless Computing is the ability to let go of the traditional way of managing servers in a company, replacing it with automated management by the cloud provider.
This means that the cloud provider is responsible for managing all organizational resources during the execution of a particular activity, leaving behind the old administrative action carried out by users within the organization.
Under this new scheme, a company’s IT activities are billed according to the need for resources for each particular task, thus creating a clear contrast with the old model where often unused spaces were hired: this allows for major capital savings, since the company only pays for what is actually used.
In addition to the above, the Serverless Computing model eliminates the need to make server reservations. As a result, developers no longer need to access the server through an Application Programming Interface (API) to add resources, since the cloud provider is now responsible for doing this automatically.
Advantages
Serverless Computing has a number of advantages when compared to the traditional model, including the following:
- It significantly reduces developer operating costs by allowing developers to pay only for used space.
- Higher productivity for companies, with the possibility to assign tasks related to the administration of servers to third parties, and thus focus directly on application development.
- Serverless Computing platforms reduce the time associated with marketing, since developers will have the option of gradually modifying or adding code.
- Providers of this new service can manage everything related to code scaling under real demand.
- Ability to focus on unifying software development and its operational capacities, that is, adopting “DevOps” system engineering practices.
- Optimized application development incorporating essential components of the BaaS model offered by other providers.
Disadvantages
Regarding the disadvantages or downsides of Serverless Computing, the following may be mentioned:
- Significant restriction on the interactive capacity of cloud providers, directly affecting system customization and flexibility.
- Dependence on service providers.
- It could cause some problems associated with the lack of control of the company’s own servers.
- Access to virtual machines and operating systems is limited.
- Implementing a serverless architecture implies an economic effort, since it typically requires updating the systems to meet the provider’s demands.
What role does the cloud provider play in Serverless Computing?
Cloud providers play a fundamental role in serverless architecture, since they are in charge of running the servers and allocating resources for developers at the same time.
In this sense, cloud providers offer two main methods within the Serverless Computing scheme, called “Function as a Services” (FaaS) and “Backend as a Services” (BaaS).
The first method, “Function as a Services” (FaaS), allows developers to apply micro services when writing and updating different codes to be implemented in the cloud, thereby simplifying the incorporation of data, reducing execution times, as well as ensuring a timely management of the supplier.
On the other hand, the “Backend as a Services” (BaaS) method is based on the provision of services to third parties based on the Application Programming Interface (API) established by the provider, such as databases, authentication services, and encryption processes.
Finally, it is worth noting that large cloud providers work under the “Function as a Services” (FaaS) mode, such as AWS Lambda from Amazon, Azure Functions from Microsoft, IBM Cloud Functions and Google Cloud.
Conclusion
Serverless Computing has certainly had a significant impact in the world of computing, allowing developers to focus on creating software without having to worry about the application management or production code, since the cloud provider is in charge of efficiently managing the resources necessary for this important activity.
Would you like to learn more about this subject? Please visit our IT Continuity page to learn more about the services we offer related to infrastructure and custom Software Development.
by Huenei IT Services | Dec 30, 2020 | Software development
In previous articles, we went through an “Introduction to Business Blockchain” and summarized the “Technical Characteristics of Blockchain“. In this latest installment of the series, we will focus on the most widespread usage patterns and analyze a flagship case of Supply Chain, aside from Decentralized Finance (DeFi).
Use cases
When analyzing the use that is being given to Blockchain in the corporate sphere, it is possible to detect some common and recurring usage patterns:
Banking and Finance
DeFi includes digital assets, protocols, smart contracts, and Distributed Applications (dApps). It is the original use case and it involves everything related to cryptocurrencies and the financial world in general. We can mention Ripple, a global network of electronic payments, with the support of institutions such as Santander, Itaú, American Express, among others. Another example is Santander One Pay FX, a Blockchain network to streamline international transfers.
Supply Chains
After DeFi, it is the most popular use case. Blockchain allows the complete traceability of any good, from the producer to the final consumer, be it raw materials, food or medicine, in the case of Pharmacovigilance. IoT (Internet-of-Things) devices are also commonly used for automated registration in different stages of a workflow. There are already numerous success stories in several industries, such as food (Walmart and later IBM Food Trust is the most emblematic cases that we will analyze), pharmaceuticals (Novartis), automotive (Ford, BMW, Tesla), among others.
Audit
Leveraging immutability, one of the distinctive characteristics of Blockchain, stored transactions cannot be modified at a later stage, which allows a complete audit of critical information. This is used in Fraud Prevention, Claims Management, Insurance (BBVA), Health (EHR, Medical Records Management with projects such as MedicalChain & MedRec) and Pharmacovigilance (for example, the Pharmaledger project).
Public Administration
Currently, many governments around the world are conducting research on how to take advantage of the benefits of Blockchain in Citizen Identity systems, voting, budgets, and public tenders to increase the efficiency and transparency of the State. Latin America strives not to be left behind, and many developments are already underway. Argentina has several projects on the Argentine Federal Blockchain network (BFA), such as sessions of Deputies in Congress, Central Bank Complaints Registry, Citizen Files in the City of Buenos Aires, among others. Brazil, Peru, and Uruguay are also making strides. Globally, the United Arab Emirates, especially Dubai, plans to become a fully Blockchain-governed, paperless city by 2021 through the Smart Dubai project.
Certified Information
Many institutions use Blockchain for the certification and validation of all types of workforce, personal and educational records (Citizen Files in Buenos Aires).
Data Sharing
In a new human-centered model, people take control of their information, centralize it to decide who can access their data. For example, patients own their complete Medical Records and can give access to them to the professional who needs them (EHR MedicalChain & MedRec), citizens can share their credentials (Sovereign Digital Identity projects in Argentina, Data Sharing Toolkit in UAE, etc.)
Asset Tokenization
Blockchain is used for the management of digital assets, in the exchange of all kinds of goods between individuals or entities, such as tickets to shows (UEFA & Ticketmaster), loyalty program points (American Express), real estate (UK Land Registry in England), etc.
Copyright
This technology is used to create records with date, time and authorship, to optimize the management of Intellectual Property. Used by Kodak with Photo Tracking and Spotify Mediachain to accurately attribute songs to creators.
Use Case: Food Supply Chain
Among the many use cases of Blockchain, its application in a supply chain is one of the most emblematic, and developments can be seen in all industries.
In a food supply chain, multiple actors have involved: farmers, ranchers, suppliers, cooperatives, packers, transporters, exporters, importers, wholesalers, retailers, and, lastly, the final consumer. Health safety is one of the biggest concerns in the food industry. Like the pharmaceutical industry, the food sector faces increased regulatory pressure from government agencies.
Walmart is a pioneer in this field, having tried several times to create a system that allows for transparency and complete traceability in the food system, which was finally accomplished in 2016. Blockchain, with its decentralized and shared ledger, seemed tailor-made for the Company’s needs. Walmart began working with its technology partner IBM on a food traceability system based on Hyperledger Fabric. For the Chinese pork industry, it allowed uploading certificates of authenticity, which gives more confidence to a system where certificates used to be a serious problem. For mangoes in the United States, the time needed to trace their origin went from 7 days… to 2 seconds!
The newly developed system allows users to know the exact origin of each item (to control disease outbreaks) in seconds, discarding only products from the affected farms. For example, it allows customers to scan a jar of baby food to see where it was made, tracing all the ingredients back to the farms.
As a result, the IBM Food Trust was launched, involving multiple companies such as Nestlé and Unilever. This platform allows access to the following information in real-time:
- Inventory at each location.
- The freshness of each product.
- Average time on the shelf.
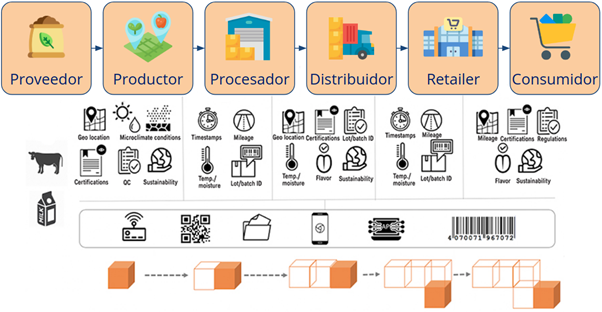
Blockchain allows a product to be traced through the different industrial, logistical, and administrative operations, from the beginning of the process to the end, and vice versa. In this way, a secure and distributed record can be consolidated with the history of every actor in the chain, their exchanges during the production and distribution processes of the product, managing information in a reliable and tamper-proof manner. As automatic transactions have no intermediaries (such as banks), they allow for faster settlements under conditions set forth in smart contracts.
IoT and Blockchain combined offer great benefits. Sensors can capture a variety of data in manufacturing facilities or transportation, transmitting all the information to a centralized repository in real-time. In turn, Managers can gain a multitude of new insights into material usage, transport conditions, etc., and apply them in planning/optimization efforts. Producers can use IoT to register the entire growth process of the product (food, pesticides, humidity, storage, location). Carriers can automatically ensure that products are moved under the right conditions of temperature, humidity, etc., thus achieving better visibility into overall logistics.
Conclusions
Throughout this 3-articles series, we learned about Blockchain technology and its application in the business environment. We were able to understand its basic operation, the most prominent platforms and its current application in many industries.
How can Huenei help your business with Blockchain?
- Consultancy: We help you choose the technology that best suits your needs.
- Architecture: Definition, deployment, and start-up.
- Development: Smart contracts and complete systems based on Blockchain.
We work with you from the planning and definition of requirements to the start-up of the final project.