by Huenei IT Services | Jan 31, 2023 | Process & Management
Nowadays, software and IT companies are immersed in a competitive market, having to deal with a changing environment, different levels of competition, and varied needs and problems from clients, among other difficulties that may affect sustainability. In this context, companies must be able to form and evolve from a solid structure that allows them to develop, grow and face adversity. The development of a business model is the fundamental tool to be able to sustain the company in the long term, grow and achieve a return on investment for the partners.
What is the Business Model Canvas Methodology?
A business model represents how an organization or company generates value, provides that value to customers, and obtains a certain benefit in return. It represents the structure on which the organization is born, develops, grows, and even dies.
In this sense, various methodologies are used to develop a business model. However, the Canvas methodology, explained by Alexander Osterwalder and Yves Pigneur in their book “Business Model Generation,” can be a handy tool so that technology companies can capture their strategy and business model on a simple canvas.
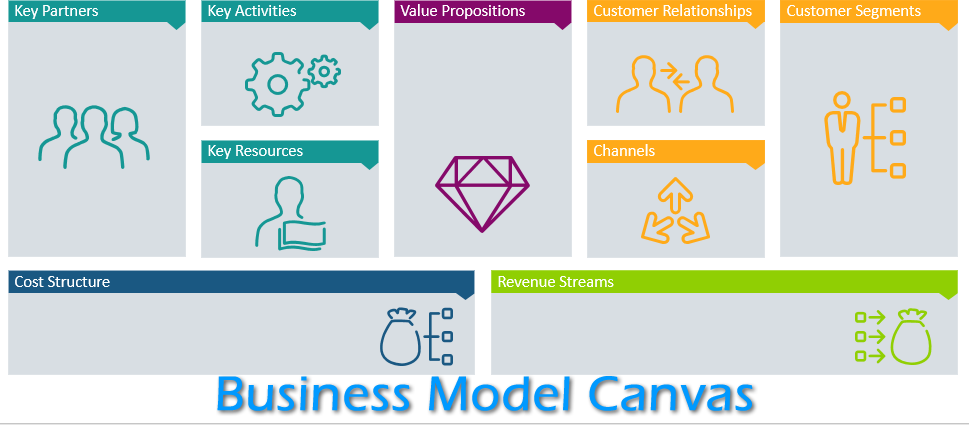
Illustration by Osmoscloud
How is the Business Model Canvas built for an IT Company?
This model is a simple canvas made up of nine quadrants that demonstrate the foundations of a technology organization’s business model. These are closely related quadrants, which leverage each other seeking a synergy that allows the organization to offer a differential to the appropriate public and obtain in return a desired benefit. The nine quadrants are built as follows:
1. Segments:
These are the public or audiences the company focuses on and serves. In this instance, it is important to make a detailed description of the individuals or organizations that make up the target segments. And it is important to understand that we can target different types of audiences, such as the following:
- Mass markets, where the objective is to attract a large number of individuals without clearly specifying the inclusion criteria for the segment. For example, the Information Technology business is characterized by a wide variety of company profiles.
- Specific segments that share a certain characteristic but still represent large volumes of individuals or organizations. An example is the Retail sector, where software products are focused on the needs of companies in the market, which represent high volumes of participation.
- Niches, characterized by a lower volume of members and a specific unsatisfied need, such as the Government segment.
- Multilateral platforms, where users of software products are two or more independent segments that interact with each other. For example, the Telecommunications, Media, and Entertainment sector is characterized by the use of software products where both the service provider company and its customers interact.
2. Value proposition:
All organizations pursue a main objective which is to satisfy the needs of their customers or solve their problems. The value proposition is the means and the tool from which we can achieve this objective. As an example, we can mention Huenei’s value proposition. We provide our clients with IT services to guide them and help them achieve their business goals through our three business units: Dedicated Teams, Staff Augmentation, Turnkey Projects.
3. Channels:
Once we determine our segments and our value proposition, it is essential to define the channels by which we will reach our customers. This is related to the communication, distribution, and sales channels that the company will use. At Huenei we rely on different communication channels, both physical and digital, and we offer our clients personalized attention throughout the project.
4. Relationship:
It is extremely important to establish strategies that allow us to build a long-term relationship with our market segments. Technology companies can provide us with very illustrative examples in terms of building relationships with clients, based on personal assistance, automated support services and co-creation in software development projects.
5. Sources of income:
The sources of income represent how the company manages to capture the value of customers. The focus at this point is on recognizing the appropriate way to capture the value of our clients through our value proposition offer. In the case of technology companies such as Huenei, the focus is usually on charging for the services and developments provided.
6. Key resources:
In order to carry out its daily operations, be it production, marketing, relationship, or others, the company needs to have certain resources. The following may be mentioned as examples:
- Physical resources, such as workplaces or points of contact with clients, programs and software used for development, and so on.
- Intellectual resources, such as patents and industrial design registrations.
- Human resources, the work team, developers, key account managers, salespeople, etc.
- Financial resources that allow the daily operation.
7. Key activities:
At this point we focus on the activities that are the foundation of the business. Those that allow the company both the generation of an attractive value proposition, as well as its contact with the public and the construction of relationships with clients. The key activities of a technology company may be related to the production or development of software products, the solution of customer problems through an after-sales support and follow-up service, the establishment of a network or platform intuitive for customers, among others.
8. Key alliances:
Sometimes, technology organizations outsource certain activities or resources important to the operation. In these cases, the partners, suppliers and allies that add value to the business and to the company’s proposal represent key players for organizational and commercial development.
9. Cost structure:
This structure is made up of fixed and variable costs incurred in the daily operations of the organization. Beyond the focus of the organization, which can be oriented towards reducing costs or increasing the value perceived by clients, the correct control and administration of the cost and expense structure of a company is essential for its survival and growth.
After this analysis of the business model structure according to the Business Model Canvas methodology, we can understand the importance of proper administration and planning of each of the quadrants for technology and software companies. As we have seen, key alliances are essential for business development, and that is why at Huenei we focus every day on offering the best service for our clients, so that they can capture that value and offer it to the segments they target. The daily work and the focus on excellence allows organizations like Huenei to collaborate in the delivery of value that companies offer to their clients.
by Huenei IT Services | Jan 30, 2023 | Process & Management
If you are an entrepreneur or a manager, then you know how hard it is to master software company management. You need to find people who have the right skills, work ethic, and personality to make your project succeed. But what if there aren’t enough developers in your area? Are there any other options? Yes! Outsourcing might be one of them.
Let’s review a series of steps that help us at Huenei to succeed in managing our business units.
Step 1: Understand the business goals and make sure that your team is aligned.
The most important thing you can do to plan your software company management is to understand the business goals and ensure that your team is aligned.
A key question is: What do you want your software team to accomplish? For example, if one project has a stated goal of improving sales by 10%, another might aim to increase revenue by 20%. This helps keep things focused on their objectives while still being able to follow up with wider issues concerning overall progress toward achieving those goals
Step 2: Choose the right structure for your company.
You should choose a team structure that is aligned with your business goals. For example, if your company is focused on developing new products and services, it makes sense to have a small team of developers working directly with the product manager.
On the other hand, if you want to scale up quickly and integrate with existing systems in fast-moving industries such as retail or banking where there are many moving parts (like web sites), then having more people involved in development will help ensure success faster than having just one person responsible for everything.
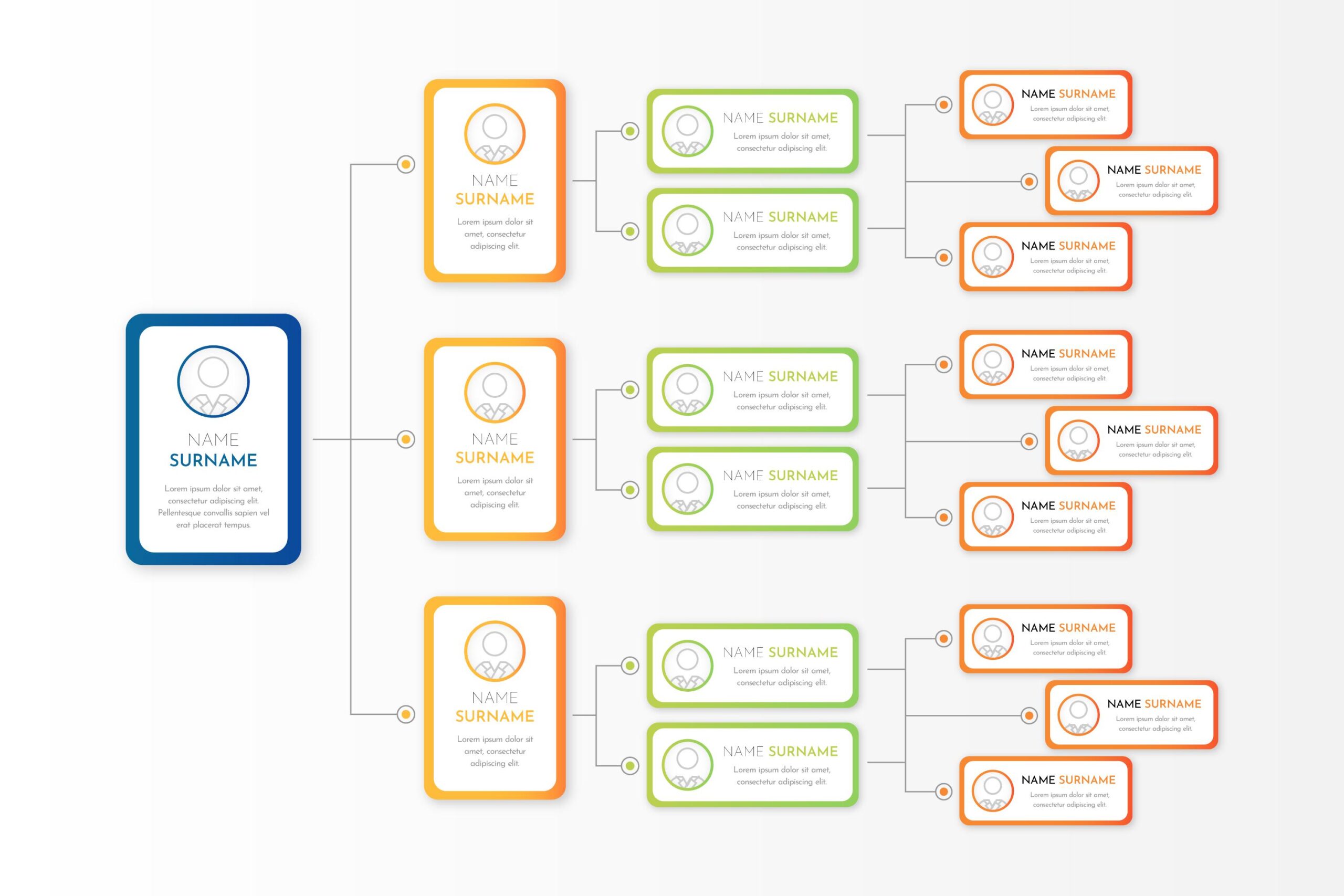
Step 3: Address team communication, feedback, and review processes.
The team structure you choose will be the foundation for your organization’s communication, feedback, and review processes. A good way to think about this is: if three different employees need to communicate with each other at any given time, how do they do it? Do they email? Do they call? Do they meet in person? If one of them is on vacation or not available at that moment, how does that employee know what’s going on in the other two’s work areas anyway?
The answer is clear: communication is key!
Step 4: Ensure regular outreach to stakeholders.
Stakeholders are the people who will use your software. They may include employees, customers, and partners. To ensure that stakeholders are involved throughout the development process, you should have a plan for engaging them.
Define your goals and objectives with stakeholders early on in the project lifecycle. This can help ensure everyone’s expectations are aligned.
Step 5: Formalize your Teams Charter – Checklist!
We offer you this resource that can be very useful to formalize your team’s charter. It is a checklist that we use in Huenei in this type of situation and that guides our decision-making:
- Define the scope of the project.
- Define your goals and objectives for this project.
- Define roles and responsibilities for each member of your team.
- Define timelines for milestones and deliverables.
- Identify key stakeholders and their expectations.
- Define communication protocols and channels for team members and stakeholders.
- Establish a system for tracking progress and measuring success.
- Identify potential risks and mitigation strategies.
- Define a process for addressing and resolving conflicts.
- Identify resources needed for the project and establish a plan for acquiring them.
- Establish a process for regularly reviewing and updating the team charter to ensure alignment with project goals and objectives.
- Identify and assign a designated project leader or manager.
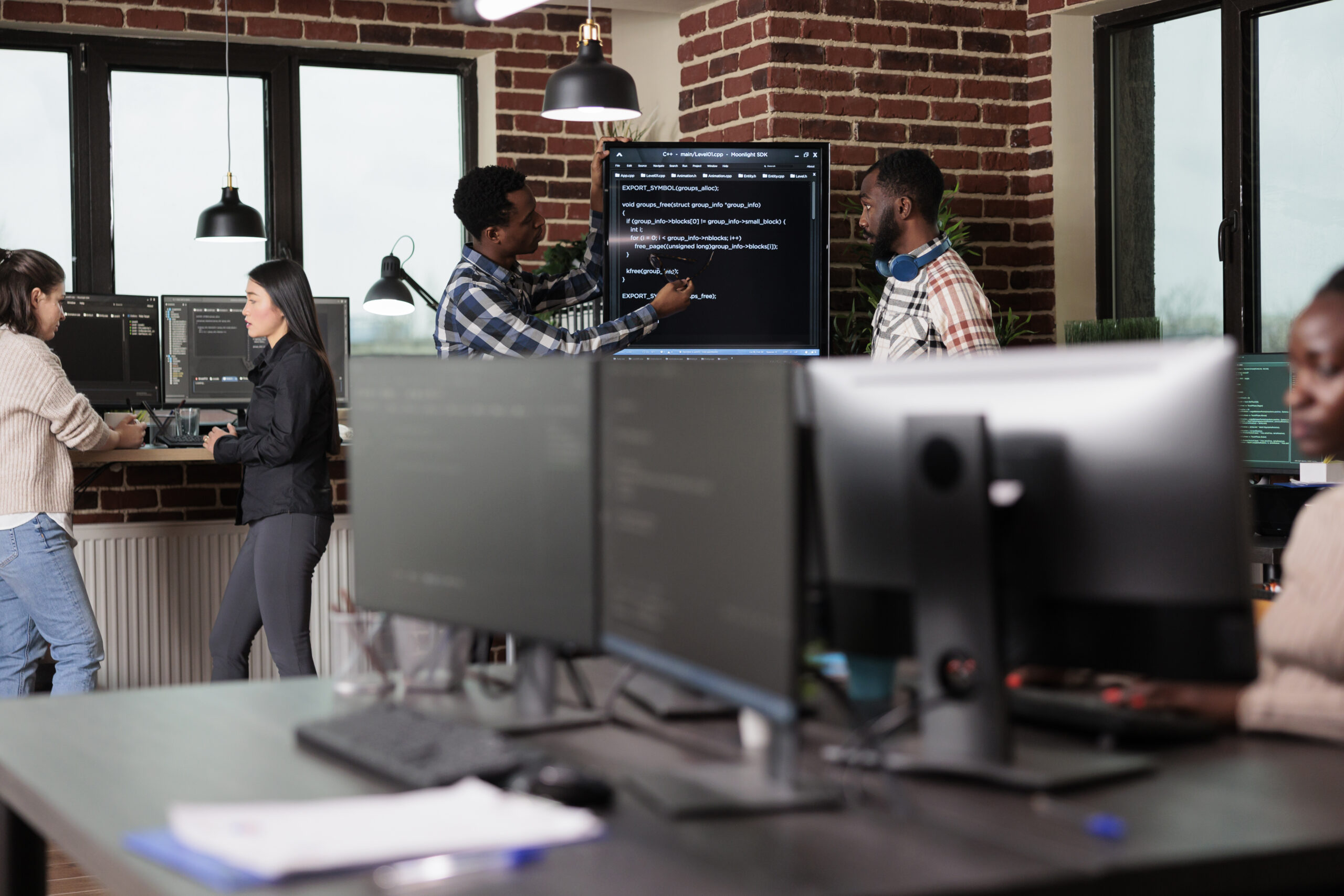
Bonus: Need more help but can’t hire new employees?
If you can’t increase your headcount, outsourcing is the best alternative. Outsourcing can be a great way to save money and time while still providing the same level of service as if you were doing it in-house.
However, before jumping into an outsourcing relationship with a new company, you must do some research and find out what kind of experience they have in this industry. You should look for a company that has experience in the same industry as yours or one that specializes in certain aspects of software development such as data science or DevOps. At Huenei we have extensive experience working on this type of project from our Agile Dedicated Teams, Application Management Outsourcing, Turnkey Projects, and Augmented Teams services.
We hope that this article has given you some insight into how to structure your software company management process. We’ve discussed different approaches and outlined some key points, but ultimately it’s up to you as an organization whether or not these ideas are right for your needs. By following through with these tips and making changes as needed over time, we believe you’ll find a successful way forward in creating a great team that can execute its goals effectively!
by Huenei IT Services | Jan 30, 2023 | Process & Management
Scrum is one of the most popular agile frameworks for project management. It’s a set of practices that help organizations get things done better and faster by ensuring they focus on what matters most.
But that doesn’t mean scrum is perfect for everyone. Many teams find it difficult to adopt this approach. This is generally the case if they don’t know where to begin or how long it will take them to succeed using the scrum methodology. So here are some tips from Huenei’s experienced scrum masters who have helped countless clients overcome their challenges!
Tip #1: Minimize your WIP. Start with one project.
First, you should minimize your work in progress (WIP). Start with one project and focus on that one thing at a time. Optimize all your processes to help you focus on that one thing.
This is a great tip for any project, but it’s especially important for Scrum projects. When you work with other people on a Scrum project, you can make the most of their ideas and contributions by focusing on one thing at a time.
For example, if someone comes up with an idea for how to improve your process or product, ask them if they want to work on that feature right now or if they want to wait until later in the project when there might be more time available. If it makes sense from both sides then go ahead and prioritize what needs doing first (or even multiple times). If not then don’t worry! Just keep working on whatever else was requested by someone else until either option comes up again later down the line.
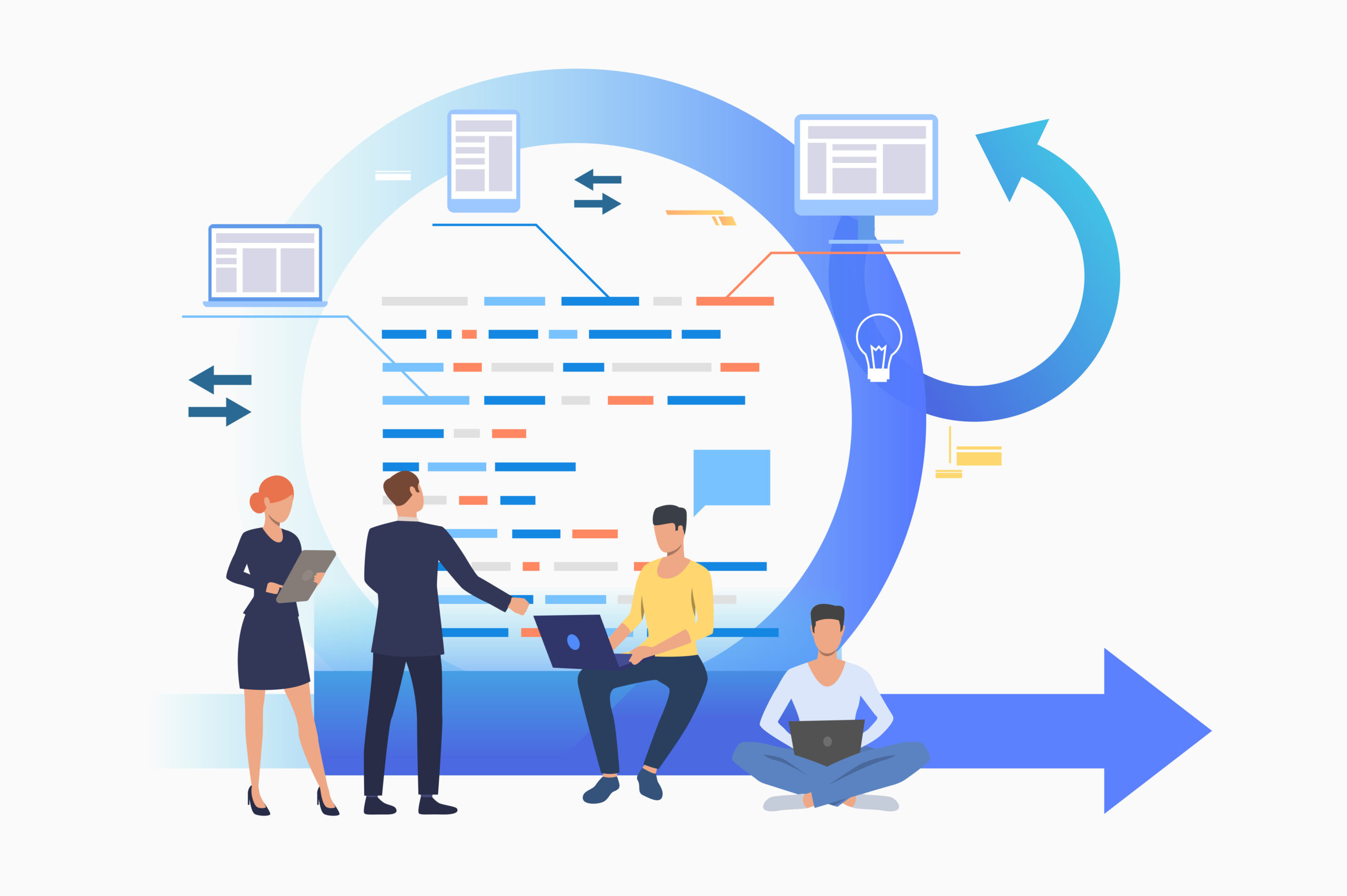
Tip #2: Optimize all your processes to help you focus on that one thing.
You can use scrum to optimize your processes by using the P1, P2, and P3 steps, that is, dividing your tasks into priorities: priority 1 (P1), priority 2 (P2), priority 3 (P3), and so on. This will help you focus on that one thing which is your software development process.
For example, if you have a lot of meetings in an organization where most people are working asynchronously, then using scrum can help you achieve better results by making sure they are all aligned with each other and have clear deadlines for when they need to be done.
Tip #3: Adapt the methodology to your needs.
We want to make sure you understand that Scrum is not a theory. It’s not a set of beliefs or ideals to be followed blindly. It’s an approach for managing your team, and there are many different ways to do it.
The official Scrum Guide is the document that defines scrum as it was originally defined by Ken Schwaber, Jeff Sutherland, and Jeff Patton in their book “Scrum: The Art of Doing Twice the Work in Half the Time.”
But if you look at this guide closely, you might find yourself reading through pages upon pages of text explaining how you should run your project! You shouldn’t worry about getting bogged down by all those details—you just need to know what they mean for your organization so that everyone knows when something goes wrong with using this method correctly.
If you try scrum and it doesn’t work well enough, keep trying something else until you succeed!
You might think that if your project is struggling, there must be something wrong with your team or process. But this is not true. There are probably a lot of things that could be causing the problem—you just don’t know what they are yet.
It’s important to remember: no matter how many times failed experiments occur, don’t get stuck on one idea! Try another one if necessary until something works better for everyone involved. You can always try again later once more information has been gathered from previous attempts at implementation.
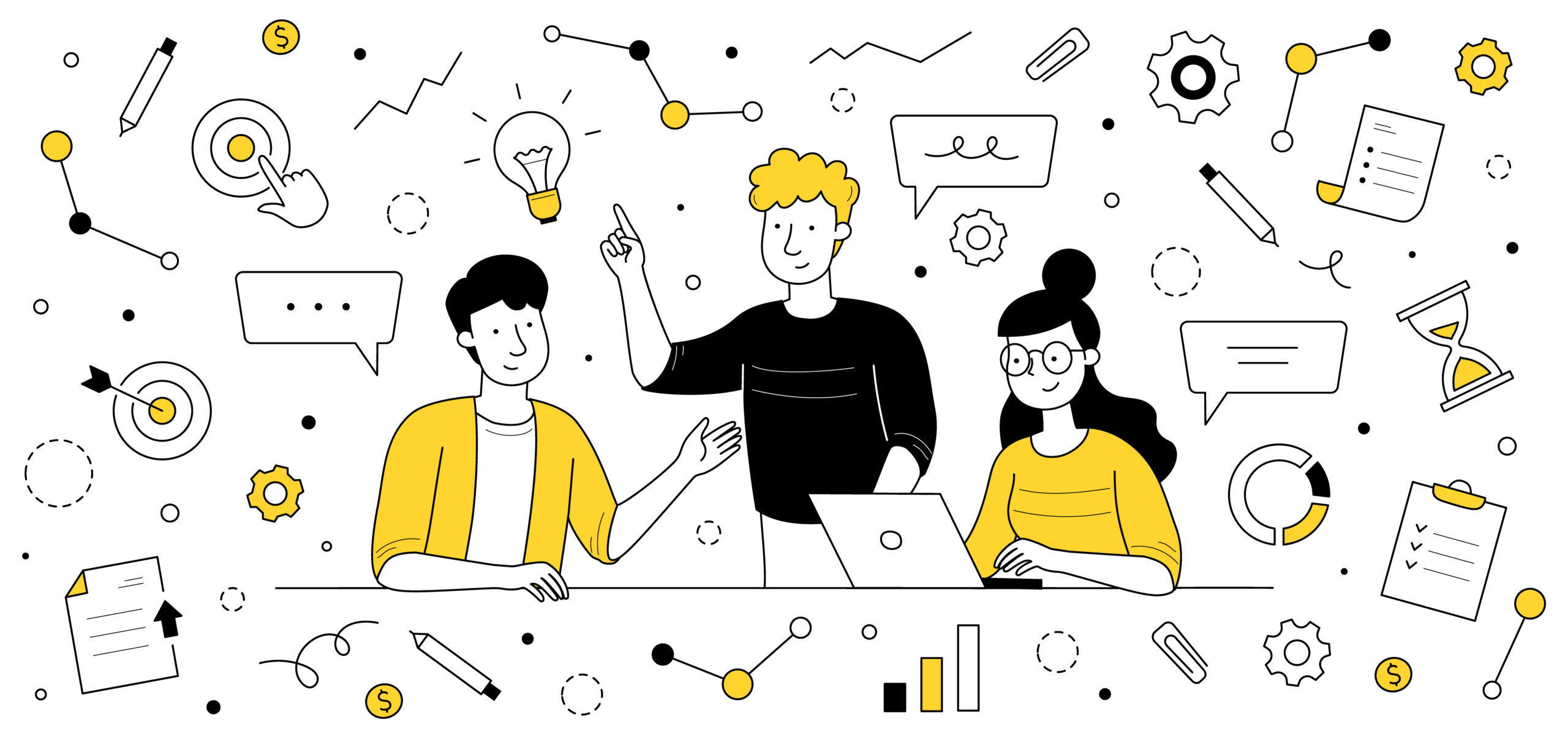
Tip #4: Keep it simple, start small, and don’t give up if it doesn’t work the first time perfectly.
When you’re working with Scrum, there are a few things to keep in mind. The first is that it’s not necessary to try and do everything at once. You can start small and work your way up as time goes on. There’s also no need to feel bad if something doesn’t work perfectly the first time—you’ll learn from your mistakes as you go along!
You should also be flexible when adapting this process. Sometimes an idea might seem like it will work but then fail when practiced in real-life scenarios like meetings or sprints. Don’t give up hope though: even if something doesn’t go according to plan initially, there are ways around most issues that come up during its use so long as they’re handled correctly by everyone involved.
All in all, Scrum is a great method for teams to improve their productivity and collaboration. It can help you manage your time better, prioritize more effectively, and be more effective at delivering value to your customers.
by Huenei IT Services | Apr 27, 2021 | Infra, Process & Management
The article Key Concepts about Data Lakes delved into the importance of Data Lakes, their architecture and how they compare to Data Warehouses. This article will focus on deployment using
Amazon Web Services (AWS), Amazon’s cloud platform. We will look into the
overall flow, the different
services available and, finally,
AWS Lake Formation, a tool specially designed to facilitate this task.
Overall Flow
Data Lakes support the needs of our applications and analytics, without the need to constantly worry about increasing storage and computing resources as the business grows and the data volume increases. However, there is no magic formula creating them. Generally, they involve dozens of technologies, tools and environments. The diagram below shows the overall flow of data, from collection, storage and processing, to the use of analytics via Machine Learning and Business Intelligence techniques.
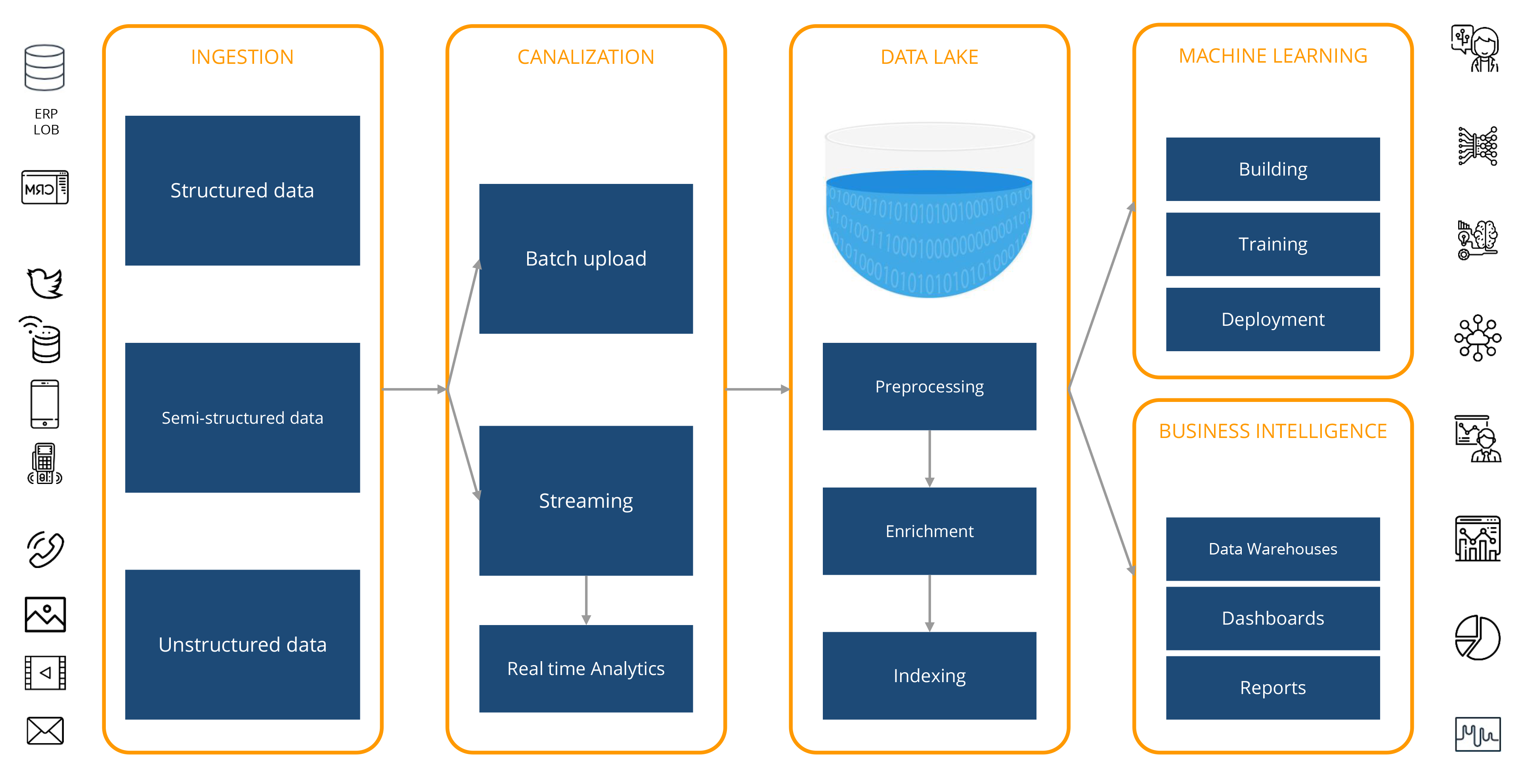
Services supported by AWS
AWS provides a comprehensive set of managed services that help build Data Lakes. Proper planning and design are necessary to migrate a data ecosystem to the Cloud, and understanding Amazon’s offerings is critical. Below are only a few of the most important tools at each stage of the flow.
Collection
The first step is to analyze the goals and benefits you want to achieve with the implementation of an AWS-based Data Lake. Once the plan is designed, data must be migrated to the Cloud, taking into account its volume. You can easily accelerate this migration with services such as Snowball and Snowcone (edge devices for storage and computing) or DataSync and Transfer Family, to simplify and automate transfers.
Channeling
In this step, you can operate in 2 modes: Batch or Streaming.
In Batch Loading, AWS Glue is used to extract information from different sources, at periodic intervals, and move them into the Data Lake. It usually involves some degree of minimal transformation (ELT), such as compression or data aggregation.
For Streaming, data generated continuously from multiple sources, such as logging files, telemetry, mobile applications, IoT sensors and social networks, are collected. They can be processed during a circular time window and channeled into the Data Lake.
Real-time analytics provides useful information for critical business processes that rely on streaming data analysis, such as Machine Learning algorithms for anomaly detection. Amazon Kinesis Data Firehose helps perform this process from hundreds of thousands of sources in real time, rather than uploading data for hours and processing it at a later stage.
Storage and Processing
The core service in any AWS Data Lake is Amazon S3, which provides high scalability storage, excellent costs and security levels, thus offering a comprehensive solution for different processing models. It can store unlimited data and any type of file as an object. It allows you to create logical tables and hierarchies from folders (for example, by year, month, and day), allowing the partition of data in volume. It also offers a wide set of security functions, such as access controls and policies, encryption at rest, registration, monitoring, among others. Once the data is uploaded, it can be used anytime, anywhere, to address any need. The service supports a wide range of storage classes (Standard, Smart, Rare Access), each with different capacities, recovery times, security and cost.
AWS Glacier is a service for secure archiving and backup management at a fraction of the cost of S3. File recoveries can take from a few minutes to 12 hours, depending on the storage class selected.
AWS Glue is a managed ETL and Data Catalog service that helps find and catalog metadata for faster queries and searches. Once Glue points to the data stored in S3, it analyzes it using automatic trackers and records its schemes. Glue is designed to perform transformations (ETL/ELT) using Apache Spark, Python scripts and Scala. Glue has no server; therefore, there is no infrastructure configured, which makes it more efficient.
If the contents of Data Lake need to be indexed, AWS DynamoDB (NoSQL database) and AWS ElasticSearch (text search server) can be used. In addition, by using AWS Lambda features, activated directly by S3 in response to events such as uploading new files, processes can be triggered to keep your Catalog up to date.
Analytics for Machine Learning and Business Intelligence
There are several options for massive Data Lake information.
Once data has been catalogued by Glue, different services can be used in the client layer for analytics, visualizations, dashboards, etc. Some of these are Amazon Athena, an interactive serverless service for ad hoc exploratory queries using standard SQL; Amazon Redshift, a Data Warehouse service for more structured queries and reports; Amazon EMR (Amazon Elastic MapReduce), a managed system for Big Data processing tools such as Apache Hadoop, Spark, Flink, among others; and Amazon SageMaker, a Machine Learning platform that allows developers to create, train and implement Machine Learning models in the cloud.
With Athena and Redshift Spectrum, you can directly query the Data Lake in S3 using the SQL language in the AWS Glue Catalog, which contains metadata (logical tables, schemes, versions, etc.). The most important aspect is that you only pay for the queries executed, depending on the scanned data volume. Therefore, you can achieve significant performance and cost improvements by compressing, partitioning, or converting data into a column format (such as Apache Parquet), as each of those operations reduces the amount of data Athena or Redshift Spectrum should read.
AWS Lake Formation
Building a Data Lake is a complex, multi-step task, including:
- Identify sources (Databases, files, streams, transactions, etc.)
- Create the necessary buckets in S3 to store data with the applicable policies.
- Create the ETLs that will carry out the necessary transformations and the corresponding administration of audit policies and permits.
- Allow Analytics services to access Data Lake information.
AWS Lake Formation is an attractive option that allows users (both beginners and experts) to immediately start with a basic Data Lake, eliminating complex technical details. It allows real-time monitoring from a single point, without having to go through multiple services. One strong aspect is cost: AWS Lake Formation is free. You will only be charged for the services you invoke from it.
It allows loading from various sources, monitoring flows, configuring partitions, enabling encryption and key management, defining transformation jobs and monitoring, reorganizing data in column format, configuring access control, eliminating redundant data, relating linked records, gaining access and auditing access.
Conclusions
These 2 articles looked into the definition of Data Lakes, what makes them different from Data Warehouses and how they can be deployed on the Amazon platform. CTO can be significantly reduced by moving your data ecosystem to the cloud. Suppliers such as AWS add new services continuously, while improving existing ones and reducing costs.
Huenei can help you plan and execute your Data Lake initiative in AWS, in the process of migrating your data to the cloud and implementing the analytics tools necessary for your organization.
by Huenei IT Services | Mar 26, 2021 | Infra, Process & Management
Data has become a vital element for digital companies, and a key competitive advantage. However, the volume of data that organizations currently have to manage is very heterogeneous and its growth rate is exponential. This creates a need for storage and analysis solutions that offer scalability, speed and flexibility to help manage these massive data volumes. How can you store and access data quickly while maintaining cost effectiveness? A Data Lake is a modern answer to this problem.
This series of articles will look into the concept of Data Lakes, the benefits they provide, and how we can implement them through Amazon Web Services (AWS).
What is a Data Lake?
A Data Lake is a centralized storage repository that can store all types of structured or unstructured data at any scale in raw format until needed. When a business question arises, the relevant information can be obtained and different types of scans can be carried out through dashboards, visualizations, Big Data processing and machine learning to guide better decision-making.
A Data Lake can store data as is, without having to structure it first, with little or no processing, in its native formats, such as JSON, XML, CSV, or text. It can store file types: images, audio, video, weblogs, data from sensors, IoT devices, social networks, etc. Some file formats are better than others, such as Apache Parquet, which is a compressed column format that provides very efficient storage. Compression saves disk space and I/O access, while the format allows the query engine to scan only the relevant columns, reducing column time and costs.
Using a distributed file system (DFS), such as AWS S3, allows to store more data at a lower cost, providing multiple benefits:
- Data replication
- Very high availability
- Low costs at different price ranges and multiple types of storage depending on the recovery time (from immediate access to several hours)
- Retention policies, allowing to specify how long to keep data before it is automatically deleted
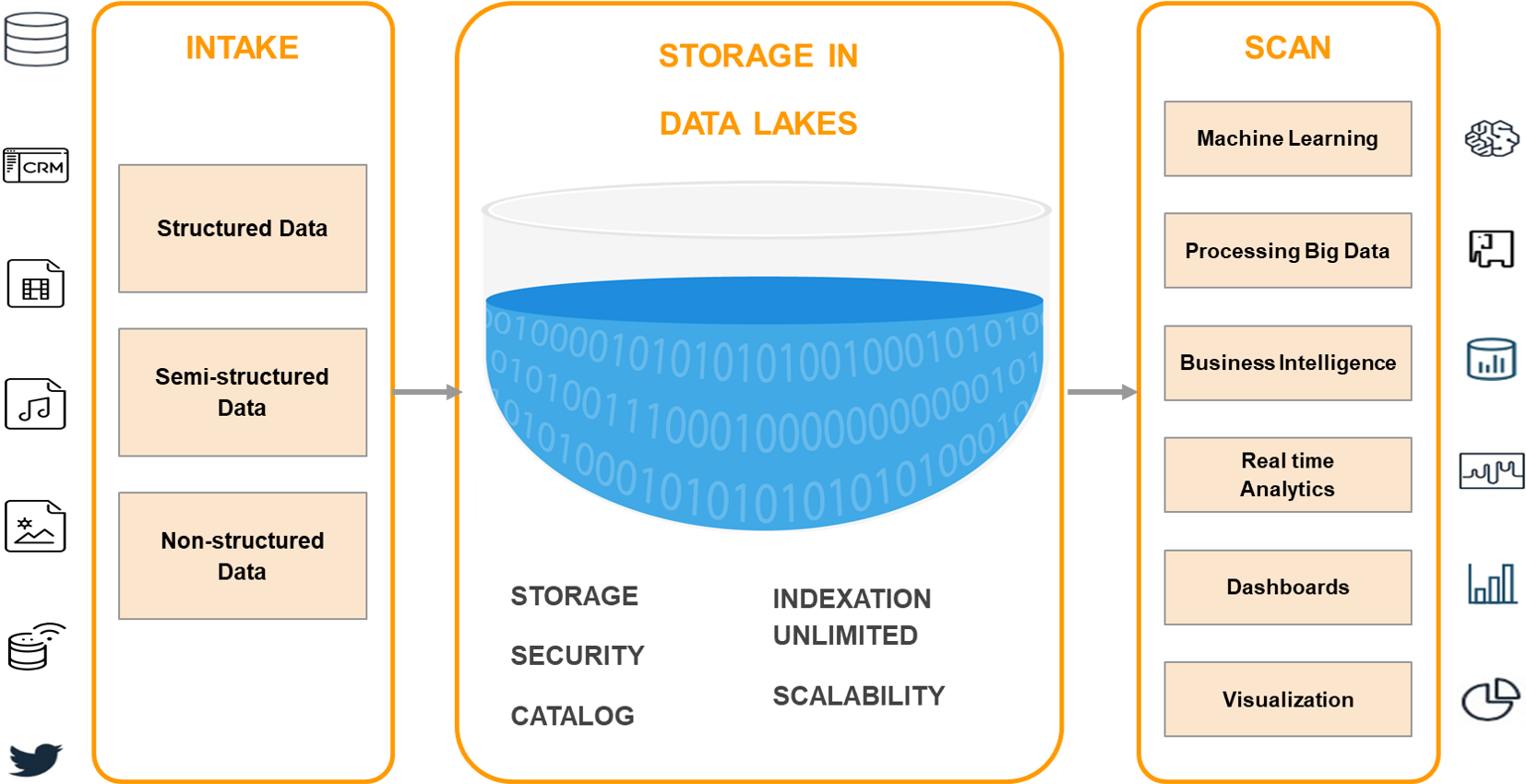
Data Lake versus Data Warehouse
Data Lakes and Data Warehouses are two different strategies for storing Big Data, in both cases without being tied to a specific technology. The main difference between them is that, in a Data Warehouse, the data scheme is pre-established; you must create a scheme and schedule your queries. Powered by multiple online transactional applications, data has to be converted via ETL (extract, transform and load) to conform to the predefined scheme in the data warehouse. In contrast, a Data Lake can host structured, semi-structured, and unstructured data and has no default scheme. Data is collected in its natural state, requires little or no processing when saved, and the scheme is created during reading to meet the processing needs of the organization.
Data Lakes are a more flexible solution adapted to users with more technical profiles, with advanced analytical needs, such as Data Scientists, since a level of skill is needed to be able to classify the large amount of raw data and easily extract its meaning. A data warehouse focuses more on Business Analytics users, to support business inquiries from specific internal groups (Sales, Marketing, etc.), by owning the data already curated and coming from the company’s operating systems. In turn, Data Lakes often receive both relational and non-relational data from IoT devices, social media, mobile apps, and corporate apps.
When it comes to data quality, Data Warehouses are highly curated, reliable, and considered the core version of the truth. On the other hand, Data Lakes are less reliable since data could come from any source in any condition, be it curated or not.
A Data Warehouse is a database optimized to analyze relational data, coming from transactional systems and business line applications. They are usually very expensive for large volumes of data, although they offer faster query times and higher performance. Data Lakes, by contrast, are designed with a low storage cost in mind.
Some of the legitimate criticism Data Lakes have received is:
- It is still an emerging technology compared to the strong maturity model of a Data Warehouse, which has been in the market for several years.
- Data Lakes could become a “swamp”. If an organization has poor management and governance practices, it can lose track of what exists at the “bottom” of the lake, causing it to deteriorate and making it uncontrolled and inaccessible.
Due to these differences, organizations can choose to use both a Data Warehouse and a Data Lake in a hybrid deployment. One possible reason would be adding new sources or using the Data Lake as a repository for everything that is no longer needed in the main data warehouse. Data Lakes are often an addition or evolution to an organization’s current data management structure rather than a replacement. Data Analysts can use more structured views of the data to get the answers they need and, at the same time, Data Science can “go to the lake” and work with all the raw information as necessary.
Data Lake Architecture
The physical architecture of a Data Lake may vary, since it is a strategy applicable by multiple technologies and providers (Hadoop, Amazon, Microsoft Azure, Google Cloud). However, there are 3 principles that make it stand out from other Big Data storage methods, and they make up its basic architecture:
- No data is rejected. They are loaded from multiple source systems and preserved.
- Data is stored in an untransformed or nearly untransformed condition, as received from the source.
- Data is transformed and a scheme is adapted during analysis.
While information is largely unstructured or geared to answering specific questions, it must be organized as to ensure that the Data Lake is functional and healthy. Some of these features include:
- Tags and/or metadata for classification, which can include type, content, usage scenarios, and groups of potential users.
- A hierarchy of files with naming conventions.
- An indexed and searchable Data Catalog.
Conclusions
Data Lakes are becoming increasingly important to business data strategies. They respond much better to today’s reality: much larger volumes and types of data, higher user expectations and a greater variety of analytics, both business and predictive. Both Data Warehouses and Data Lakes are intended to coexist with companies that want to base their decisions on data. Both are complementary, not substitute, and can help any business to better understand both markets and customers, as well as promote digital transformation efforts.
Our next article will delve into how we can use Amazon Web Services and its open, secure, scalable, and cost-effective infrastructure to build Data Lakes and analytics on top of them.