Discover the 7 key metrics to effectively improve code quality, reduce risks, streamline processes, and optimize results.
Results for "Software development":
Scaling Agile Methodologies
Discover how companies can scale agile methodologies using frameworks like SAFe and LeSS to maintain productivity and alignment.
Managing Technical Debt: Impact on Scalability
Managing technical debt is essential for scalability, smooth cloud migration, and preparing systems for AI. Discover strategies and tools.
How can Information Systems Help your Business?
Maximize business efficiency with Information Systems. Explore how strategic integration can elevate your operations and drive sustainable growth.
Software Outsourcing as an Alternative to Leverage your Business
Outsourcing is a business solution based on transferring resources and responsibilities to another company dedicated precisely to the provision of those services. In the world of information technology, software outsourcing has become an increasingly common practice,...
Minimum Viable Product Examples and Overview
If you think that building a product only after you have started selling it to people is a mad idea, we are here to show you great minimum viable product examples that prove the opposite. MVPs can be any ideas or products that feature only a limited set of functions...
Best Code Review Practices: Improve your Coding like a Pro
Do you want to avoid problems in software development? You may need to know what the best code review practices are. In this case, we will tell you which strategies are necessary for you to understand how to program in the best possible way. The importance of knowing...
Coding in Python: Tips and Tricks for your Software Development.
Are you learning to program and need some help? Don't worry: we've all been there. In this case, we will tell you which are the best ideas for coding in Python. We assure you that, after reading this article, you will have better references to make your work routine...
How to scale up your tech startup with software development outsourcing.
How to scale up your tech startup with software development outsourcing. The continuous growth of your startup can be both exciting and scary at the same time. This is what all tech companies dream of, but growth does not come easy. If building a tech startup is...
The Complete Guide to Rapid Application Development
Have you ever been interested in improving your company's software development? Well, you may be able to take advantage of some interesting methodologies to increase the efficiency, productivity, and quality of your deliveries, and one of them could be Rapid...
What is a Software Factory and Why You Should Hire One
Organizations today must develop technological solutions capable of responding quickly and efficiently to customer demands, and thus offer the best experiences. This new digital paradigm gave rise to the birth of software factories. Software currently plays an...
A Few Use Cases for Serverless Computing
Introduction The revolution of Serverless Computing is here to stay, and this is because this new technology enables application development without having to go through the management and administration of a server. Under this model, applications can be grouped and...
Let's get there, together
Thank you for visiting our website! We value your interest on us and we would love to hear from you. If you have any questions or comments, please don't hesitate to get in touch. We'll contact you asap to help you!
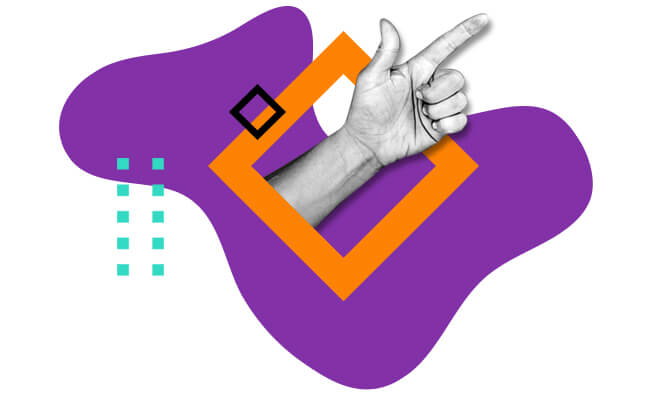